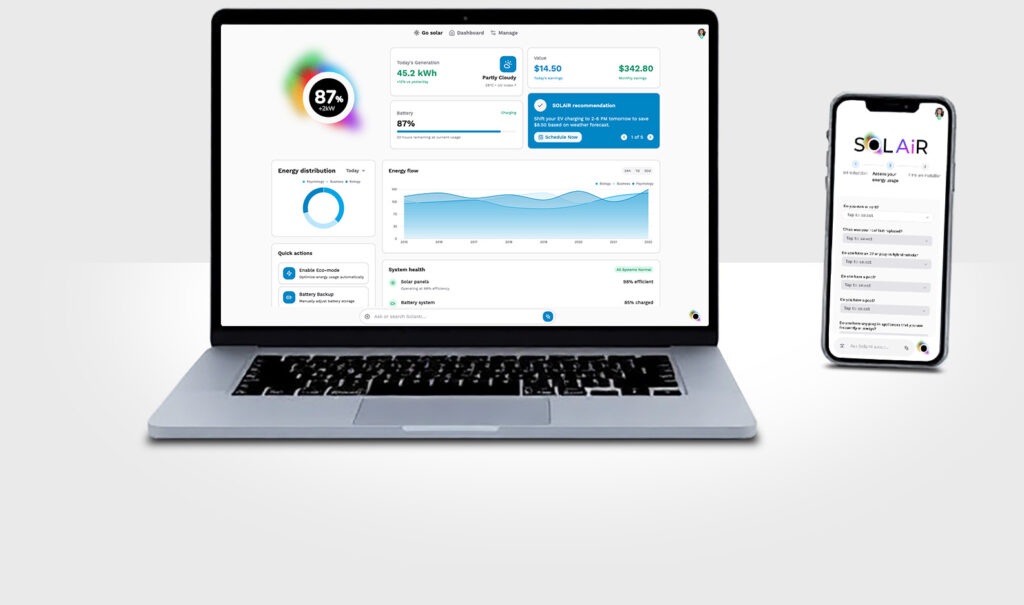
AI Solar bidding assistant – automating solar adoption through AI
Or: How I learned to leverage AI to reduce solar installation costs, eliminate friction, and accelerate adoption
Role: AI product design | UX strategy | Emerging tech leadership
Key focus areas
- Customer Acquisition Cost reduction
- AI-driven proposal automation
- User-Centered energy optimization
What this case study covers
- How AI product design solves real-world sustainability challenges
- From research to prototype: My process in defining, designing, and testing AI-driven solutions
- My role in leading UX, product vision, and AI integration
“People want to save the planet,
but they demand to save money.”
~ Josh Plaisted, expert solar consultant
Key challenges in solar adoption
Solar energy is still too expensive for many homeowners, and they are uncertain if the investment will be reflected in the resale value of the home. While hardware costs have dropped significantly, customer acquisition and installation processes remain outdated an expensive.
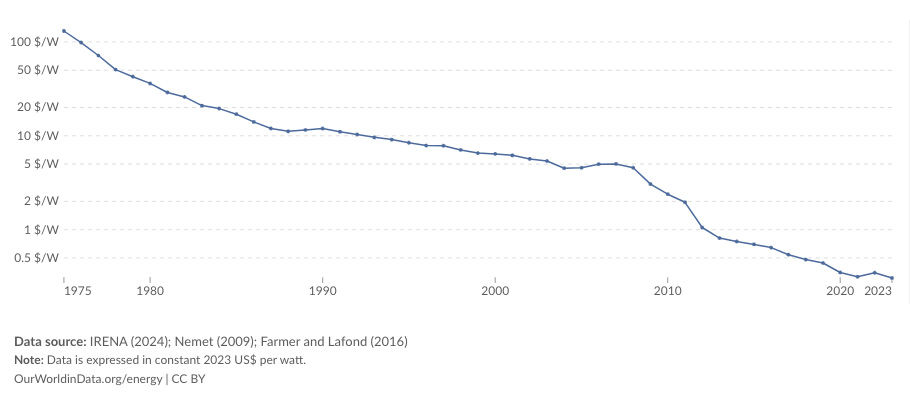
The root problem: Customer Acquisition Cost
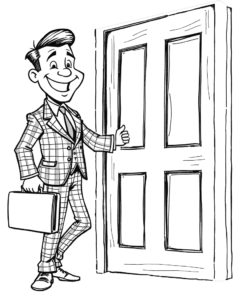
- Solar installation costs ~$4 USD/watt in North America, compared to $1.75 USD/watt in Australia.
- 33% of solar costs are from sales and proposal generation.
- Manual processes (door-to-door sales, in-person assessments) create latency and friction in decision-making.
Customer perspective
- Homeowners are interested in solar but overwhelmed by the process.
- Too many variables: grid buyback rates, installation logistics, energy efficiency optimization.
- They need clear, actionable information to make informed decisions.
Business perspective
- Sales teams rely on expensive, slow lead-generation tactics.
- Customer engagement is low due to cognitive overload and complex onboarding.
Opportunities for AI automation
Do you find yourself asking:
- Can AI automate and simplify the entire solar bidding process?
- Can we eliminate the need for door-to-door sales?
- Can we help users make energy-smart decisions effortlessly?
No? Then you’re not alone. That’s why I’ve put together this case study!
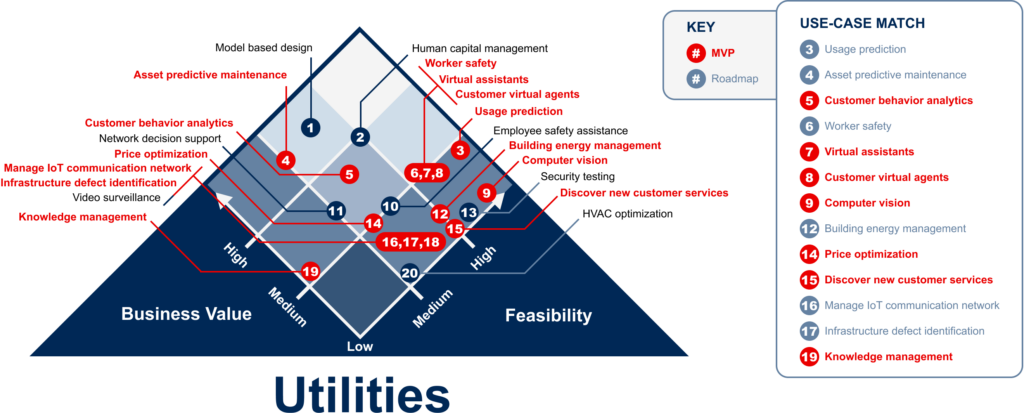
Referencing Gartner’s use-case prism for Utilities sector – I identified 7 key touch points for AI automation in an MVP product, with an additional 6 use-cases for future roadmap planning.
“Just the facts, ma’am”
~ A colloquial misquote of Sgt. Joe Friday, Dragnet
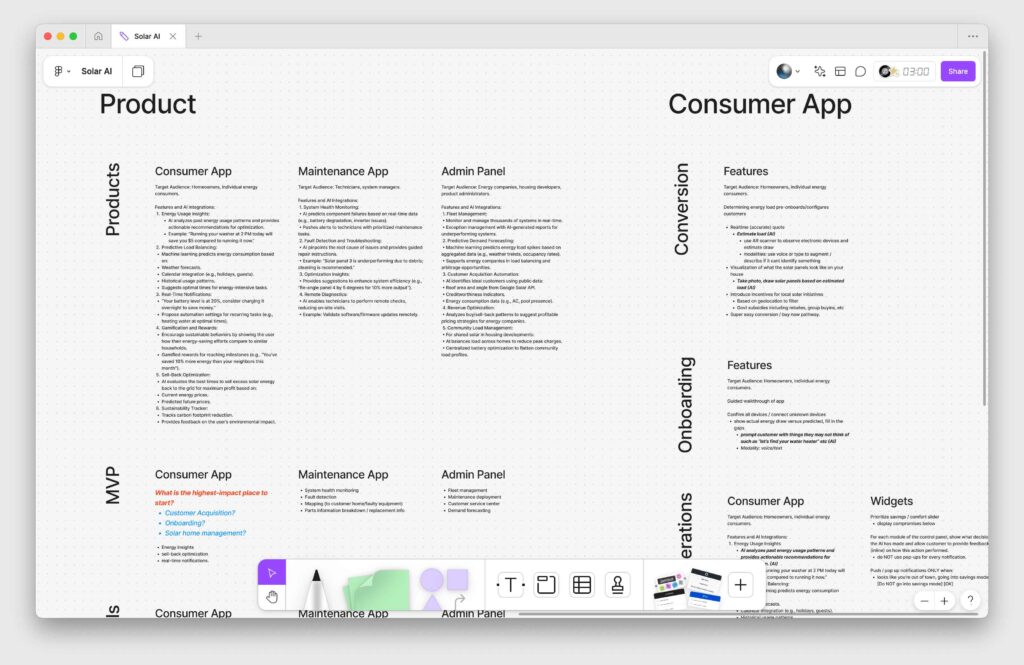
Research methods
- Expert interviews – Solar industry professionals, AI engineers
- Competitive analysis – Existing tools like Fluence, Bidgely, Sense, Josh.ai, etc.
- User insights – From solar homeowners to solar-curious homeowners, pain points in solar adoption, cognitive barriers
Findings from expert interviews
- Customer acquisition is the #1 cost factor. Door-to-door sales aren’t scalable.
- People want to save the planet, but they DEMAND to save money.
- Current solar apps are made for engineers, not everyday homeowners.
- Automating proposal generation could cut onboarding time by 75%.
Competitive gaps identified
- Existing platforms don’t simplify onboarding.
- Very few AI tools exist to intelligently match users to optimal solar configurations.
- Consumers have no central hub for researching, bidding, and managing solar systems.
Key insight 💡 AI can bridge the knowledge gap
By leveraging multimodal AI (Computer vision, chatbot UX, and predictive analytics), we can simplify the process and empower users.
The solution: AI Solar proposal assistant

A multimodal AI-powered assistant that:
- Educates users on solar feasibility via AI chatbot
- Uses computer vision to analyze rooftops, estimate installation feasibility
- Generates automated proposals with cost breakdowns, best financing options
- Predicts energy load and ROI for users based on real-time energy data
- Integrates with installers for seamless bid management
How AI solves the problem
- Step 1: AI chatbot answers customer questions in natural language
- Step 2: Homeowners scan appliances with their phone → AI estimates energy consumption
- Step 3: AI generates customized solar proposal based on home data & usage patterns
- Step 4: User gets auto-generated quotes from multiple solar providers
- Step 5: AI assists in energy optimization post-installation
Outcome: Reducing solar cost by 33%, eliminating friction, and accelerating adoption.
AI Implementation & UX considerations
AI modalities used
- Computer Vision → Identify roof conditions, appliances, energy consumption
- Conversational AI → Natural language assistant to guide users
- Automated Bidding AI → Instantly matches users with local installers
- Predictive AI → Forecast ROI, savings, grid buyback potential (future scope)
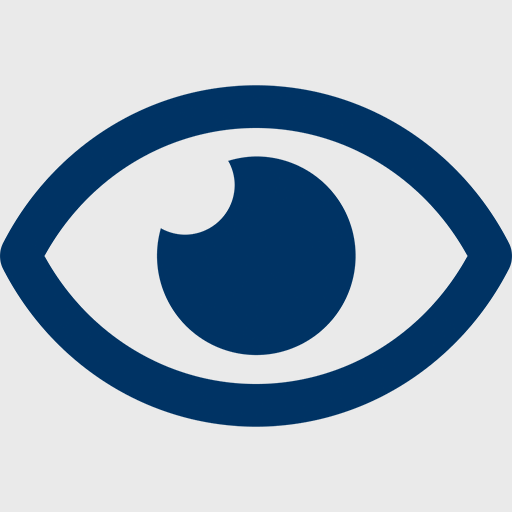
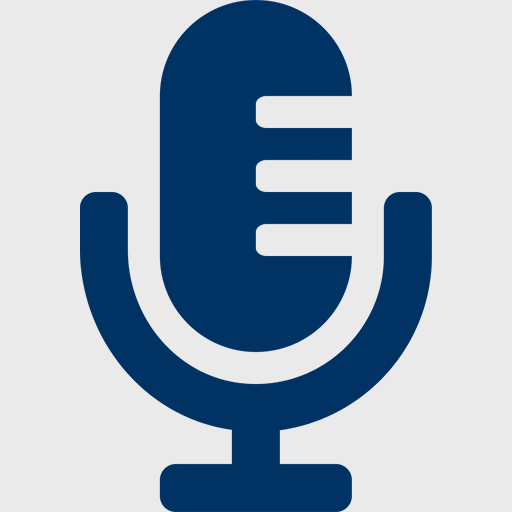
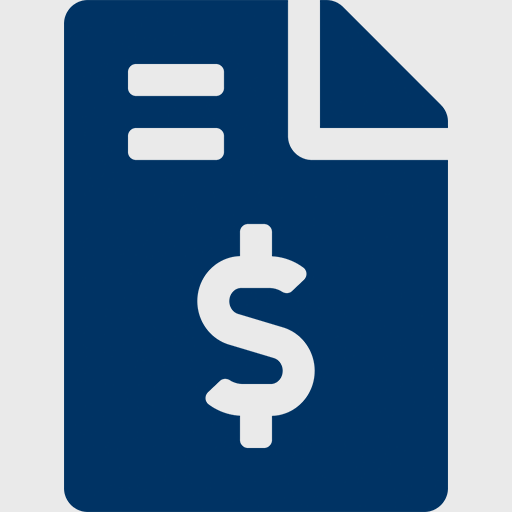
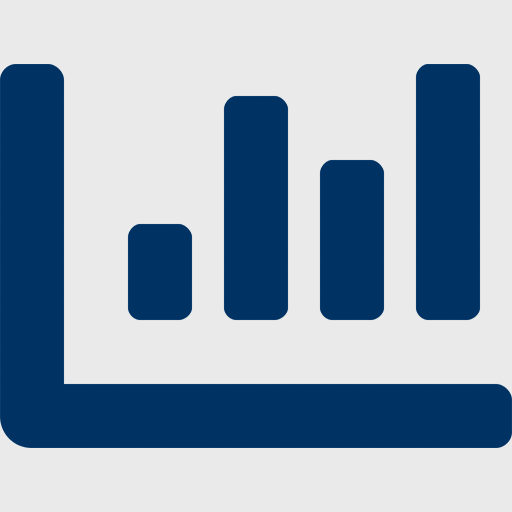
UX challenges & solutions
❌ Problem: Users fear over-automation & lack of control
✔ Solution: Explainable AI → Transparency in how estimates are generated
❌ Problem: AI recommendations might not always be accurate
✔ Solution: User validation checkpoints → Allow manual adjustments
Measuring impact
Much of this product relies on a human factor of process-change, which will take time and real-world feedback to gauge effectiveness. Local installers will need to learn to trust incoming bids from SOLAiR.
Estimated success metrics
- ✅ Customer Adoption → Reduced onboarding friction → 30% more engagement
- ✅ Cost Reduction → AI eliminates sales labor → 33% lower acquisition costs
- ✅ Faster Conversion → Instant proposals → 50% faster quote-to-contract cycle
- ✅ Energy Optimization → AI-assisted recommendations → 10-15% lower household energy costs
Expected business outcomes
- Faster, cheaper solar installations → more users converting
- Lower cost = wider adoption → accelerates clean energy transition
Key learnings & next steps
What I learned
- AI is only useful if it aligns with human needs.
- An AI chatbot is not an end-all solution. AI modalities need to respond to the context of the situation to empower the customer to accomplish their goal.
- Trust & transparency are critical in AI-driven decision-making.
- Users want automation, but not full control loss → UX must balance AI autonomy & user input.
What’s next?
- 🚀 Testing with users → validating AI’s accuracy & usability
- 🚀 Partnering with solar companies → refining proposal automation
- 🚀 Expanding AI capabilities → smart home integrations for energy optimization
Looking for collaborators!
I’m passionate about building AI-driven sustainable products. If you’re working on AI in climate tech, UX for AI, or sustainable energy innovations—let’s connect!
📩 Want to see the prototype in action? Message me!